Linear Algebra Uncovered: Mastering Vectors and Matrices in Your Homework
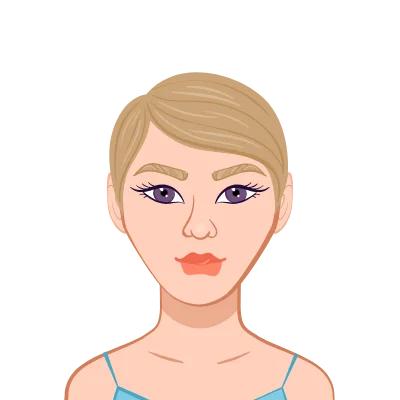
Linear algebra, a fundamental branch of mathematics, holds a pivotal role across diverse disciplines including physics, computer science, economics, and engineering, where its applications permeate through complex problem-solving endeavors. Its omnipresence renders it an indispensable tool, providing a universal language for expressing relationships and transformations. This blog post endeavors to unravel the intricacies of linear algebra, with a specific focus on vectors and matrices—two bedrock concepts that underpin the entire mathematical framework. Whether you find yourself grappling with homework assignments as a student or seek to fortify your comprehension as a seasoned professional, this comprehensive guide aspires to demystify the often intimidating terrain of linear algebra. By delving into the core principles and applications of vectors and matrices, we aim not only to elucidate their theoretical underpinnings but also to equip you with the knowledge and confidence to navigate and conquer mathematical challenges with assurance. This exploration, aimed at solving your Math assignment, will not only facilitate a deeper understanding of the abstract concepts within linear algebra but will also empower you to wield its principles as practical tools in addressing real-world issues, thereby bridging the gap between theoretical knowledge and tangible problem-solving skills. As we embark on this journey through the intricacies of linear algebra, we invite you to explore the elegance and utility inherent in its principles, fostering a holistic appreciation for a discipline that serves as the linchpin for countless applications in our technologically-driven world. Whether you are charting your academic path or seeking to augment your professional toolkit, the insights gained from this exploration are designed to resonate, providing a solid foundation for grappling with the challenges and opportunities that linear algebra unfolds across various domains of knowledge and application.
The Building Blocks: Vectors
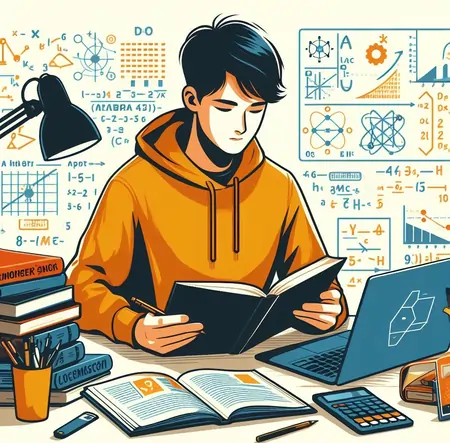
In the realm of linear algebra, vectors stand as fundamental building blocks, serving as essential entities that encapsulate both magnitude and direction. Defined as mathematical objects, vectors possess a geometric and algebraic essence, finding representation through arrows in space or ordered arrays of real numbers. The intricacies of vector operations, encompassing addition, subtraction, and scalar multiplication, unveil the mathematical ballet underlying the manipulation of these entities. Furthermore, the concept of vector spaces emerges, elucidating the foundational properties that govern sets of vectors and their interactions within a given mathematical framework. As students delve into the world of vectors, geometric interpretations intertwine with algebraic manipulations, paving the way for a comprehensive understanding of these mathematical entities. Whether visualizing vectors in two or three dimensions or comprehending their roles in diverse mathematical applications, mastering the nuances of vectors forms the bedrock upon which a more profound comprehension of linear algebra is built. In the subsequent sections, we will unravel the intricacies of matrices, exploring their dimensions, operations, and applications, further enhancing the reader's journey into the heart of linear algebra.
Definition and Representation:
Linear algebra's cornerstone, vectors, are mathematical entities signifying both magnitude and direction. These fundamental quantities are represented geometrically and algebraically. In the geometric realm, vectors find expression as arrows in space, encapsulating both length and direction. Algebraically, vectors are represented as ordered lists of numbers, illustrating their components. Understanding these representations is crucial, laying the groundwork for navigating the complex landscape of linear algebra.
Operations on Vectors:
Vectors, as versatile mathematical entities, undergo a spectrum of operations pivotal to their manipulation and application. Addition and subtraction of vectors involve combining or separating quantities with direction and magnitude. Scalar multiplication, a fundamental operation, allows the scaling of vectors, impacting their magnitude. These operations form the backbone of linear algebra, serving as the building blocks for more advanced concepts. Real-world scenarios, ranging from physics to computer science, highlight the significance of vector operations in problem-solving and modeling.
Vector Spaces:
The mathematical realm expands with the concept of vector spaces, defined by specific properties that govern their structure. Closure under addition and scalar multiplication, associativity, and the existence of additive and multiplicative identities characterize these spaces. Understanding vector spaces is vital, as they provide a framework for exploring linear algebra's depth. From polynomial spaces to Euclidean spaces, vector spaces offer a diverse landscape where mathematical operations unfold, guiding the exploration of complex systems and paving the way for the comprehension of more advanced linear algebra concepts.
Matrices: The Powerhouse of Linear Algebra
Matrices, often referred to as the powerhouse of linear algebra, constitute a cornerstone in the understanding and application of this mathematical discipline. Defined as rectangular arrays of numbers, matrices are indispensable tools with versatile applications across various fields. This section explores the intricate structure of matrices, elucidating their dimensions, elements, and different types. Beyond their mere representation, matrices are dynamic entities subject to a range of operations – addition, subtraction, multiplication, and scaling. These operations, discussed in detail, not only serve as the building blocks for advanced linear algebra concepts but also find practical applications in solving linear systems, representing transformations, and manipulating data. Delving into special types of matrices, such as symmetric matrices, diagonal matrices, and identity matrices, unveils their unique properties and their pivotal roles in specific mathematical contexts. With matrices serving as the linchpin of linear algebra, this section unravels their profound significance, laying the groundwork for comprehending and mastering the broader landscape of mathematical applications in science, engineering, and computer science.
Definition and Representation:
Matrices, fundamental in linear algebra, are rectangular arrays of numbers, symbols, or expressions arranged in rows and columns. Understanding their structure involves grasping dimensions, elements, and types. Matrices form the backbone for solving linear systems and representing transformations. Comprehending their definition and representation lays the groundwork for advanced applications in various fields.
Matrix Operations:
Matrices, powerful tools in linear algebra, undergo various operations crucial for solving complex problems. Addition, subtraction, multiplication, and scaling are fundamental matrix operations with applications in solving linear systems, representing transformations, and manipulating data. Examining these operations in depth provides insight into their significance and versatility across diverse disciplines.
Special Types of Matrices:
Matrices come in diverse types, each with unique properties and applications. Symmetric matrices, diagonal matrices, and identity matrices are among the special types that play crucial roles in specific contexts. Understanding their properties is essential, as these matrices simplify computations and analyses, offering efficient solutions to a wide array of problems. Delving into special matrices enriches our understanding of their relevance and impact within the realm of linear algebra.
Solving Systems of Linear Equations
Solving systems of linear equations is a pivotal application of linear algebra, with methods like Gaussian elimination serving as potent tools for finding solutions. In this critical phase of mathematical problem-solving, the focus is on transforming augmented matrices into row-echelon form through systematic elimination steps. Gaussian elimination, a widely employed technique, systematically applies elementary row operations to the augmented matrix until it reaches a simplified, triangular form that facilitates straightforward solutions to the system. The process involves pivot elements and the creation of zeros below them, gradually reducing the system to a more manageable state. Beyond Gaussian elimination, matrix inversion plays a significant role in solving linear systems. Inverting a matrix allows us to find solutions efficiently by applying the inverse to the augmented matrix. Understanding the conditions for matrix invertibility is key to employing this method effectively. Overall, the exploration of solving systems of linear equations provides a practical toolkit for tackling real-world problems, emphasizing the versatility and power that linear algebra brings to mathematical applications.
Gaussian Elimination:
Gaussian elimination, a fundamental method in linear algebra, is pivotal for solving systems of linear equations. The process involves transforming an augmented matrix into its row-echelon form through a sequence of elementary row operations. This systematic approach simplifies complex systems, making it easier to find solutions. By applying elimination steps, we can navigate through the matrix, ultimately arriving at a form where variables are easily isolated, revealing the values that satisfy the system of equations. This technique not only aids in homework problem-solving but also finds extensive use in diverse mathematical applications.
Matrix Inversion:
Matrix inversion is a key concept with broad applications in linear algebra, cryptography, and optimization. For a square matrix to be invertible, it must be non-singular, implying that its determinant is non-zero. The process involves finding the inverse matrix, such that when multiplied with the original matrix, it yields the identity matrix. Inverting a matrix enables the efficient solution of systems of linear equations, contributing to the foundation of various mathematical and computational tasks. This concept's significance extends beyond the confines of homework, becoming an indispensable tool in mathematical analyses and algorithm development.
Eigenvalues and Eigenvectors
Eigenvalues and eigenvectors, pivotal concepts in linear algebra, provide profound insights into the behavior of linear transformations and matrices. Eigenvalues represent the scaling factors by which a matrix transforms its corresponding eigenvectors. Understanding these eigenpairs unlocks the ability to analyze and interpret the inherent structure and characteristics of linear transformations. Geometrically, eigenvectors remain unchanged in direction but may undergo scaling during the transformation, making them crucial in applications such as physics, structural engineering, and quantum mechanics. In addition to their geometric significance, eigenvalues and eigenvectors play a crucial role in diagonalization, a process that simplifies matrix computations and facilitates the understanding of complex systems. Whether unraveling the dynamics of dynamic systems or optimizing algorithms in machine learning, the concepts of eigenvalues and eigenvectors form the backbone of sophisticated mathematical modeling. As we explore their properties and applications, we gain a deeper appreciation for their ubiquity across various disciplines, emphasizing their role as indispensable tools for both theoretical analyses and practical problem-solving in the vast landscape of linear algebra.
Understanding Eigenvalues and Eigenvectors:
Understanding Eigenvalues and Eigenvectors is fundamental in linear algebra, representing intrinsic properties of matrices. Eigenvalues signify the scaling factors in linear transformations, while eigenvectors denote the corresponding directions unaffected by the transformation. This concept is pivotal in various applications, such as physics and data analysis, where understanding the inherent structure of linear transformations is crucial.
Diagonalization:
Diagonalization, a powerful technique in linear algebra, simplifies the analysis of matrices by expressing them in diagonal form. This process involves finding a diagonal matrix D and an invertible matrix P such that P−1AP=D. Diagonalization has practical applications in solving systems of linear differential equations and understanding repeated applications of linear transformations, making it a valuable tool in advanced linear algebra and its diverse applications.
Applications in Computer Science and Machine Learning
In the realm of computer science and machine learning, the applications of linear algebra are ubiquitous and transformative. In computer graphics, matrices and vectors form the backbone for representing and manipulating 3D objects, allowing for the creation of realistic virtual environments and animations. The mathematical underpinnings of linear transformations, rotations, and translations are essential for rendering graphics with precision and efficiency. Transitioning into the domain of machine learning, linear algebra becomes a cornerstone in data representation and algorithmic implementation. Matrices serve as powerful tools for organizing and manipulating data, while vectors encapsulate features and attributes crucial for model training. The principles of linear algebra are deeply embedded in machine learning algorithms, facilitating tasks such as regression, classification, and clustering. From constructing neural networks to optimizing models, a profound understanding of linear algebra empowers computer scientists and machine learning practitioners to navigate the complexities of data analysis and pattern recognition, making it an indispensable skill set in the ever-evolving landscape of technology.
Linear Algebra in Computer Graphics:
Linear algebra serves as the backbone of computer graphics, providing a mathematical framework for representing and manipulating 3D objects. Matrices and vectors play a pivotal role in modeling transformations, rotations, and translations, essential for rendering realistic scenes in video games, simulations, and animations. Understanding linear algebra enables developers and graphic designers to create visually stunning and immersive digital environments by precisely defining the positions, orientations, and scaling of objects in a virtual space.
Machine Learning and Linear Algebra:
In the realm of machine learning, linear algebra is a fundamental pillar that underpins various algorithms and methodologies. Matrices and vectors are central to representing and manipulating data, allowing for efficient computation and analysis. Linear algebra provides the tools necessary for formulating and solving optimization problems, a key aspect of machine learning tasks such as regression and classification. Whether it's dimensionality reduction, feature engineering, or implementing neural networks, a solid grasp of linear algebra is essential for machine learning practitioners to design robust and effective models capable of handling complex datasets.
Conclusion:
In conclusion, the journey through the intricacies of linear algebra, focusing on the fundamental concepts of vectors and matrices, unveils a profound understanding of a mathematical realm that permeates various disciplines. From the basic operations of vectors to the transformative power of matrices, this exploration not only equips students with the tools to conquer homework assignments but also empowers professionals to tackle complex real-world challenges. The importance of linear algebra becomes evident as we delve into applications, ranging from solving systems of linear equations to its pivotal role in computer graphics and machine learning. By unraveling the mysteries of eigenvalues and eigenvectors, and the practicality of diagonalization, the reader gains a comprehensive grasp of concepts that extend far beyond the confines of a classroom. As technology advances, the applications of linear algebra continue to evolve, solidifying its status as a cornerstone in the foundation of scientific and technological endeavors. In essence, mastering linear algebra is not merely an academic pursuit but a gateway to a world where mathematical principles seamlessly integrate with the complexities of the real world, shaping solutions and innovations across diverse domains.