The Uncertainty Principle in Harmonic Analysis and Signal Processing
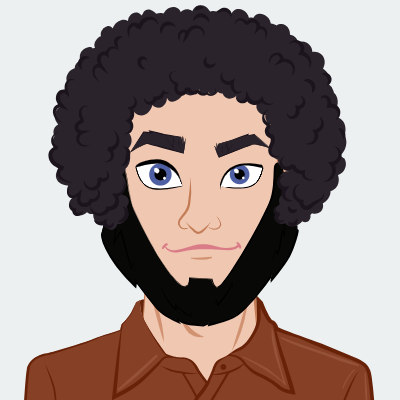
In the world of mathematics and science, there are certain principles and theorems that play a foundational role in shaping our understanding of the universe. One such principle is the Uncertainty Principle, a concept that originated in quantum mechanics but has found its way into various fields, including harmonic analysis and signal processing. In this blog, we will delve into the fascinating world of the Uncertainty Principle and explore its applications and implications in these two critical domains. So, whether you're trying to complete your geometry assignment or simply seeking to expand your knowledge, understanding the Uncertainty Principle is a crucial step in your scientific journey<
What is the Uncertainty Principle?
The Uncertainty Principle, originally formulated by German physicist Werner Heisenberg in 1927, is a fundamental concept in quantum mechanics. At its core, it states that the more precisely we know a particle's position, the less precisely we can know its momentum, and vice versa. In other words, there is an inherent limit to how accurately we can simultaneously measure certain pairs of properties of a particle, such as position and velocity.
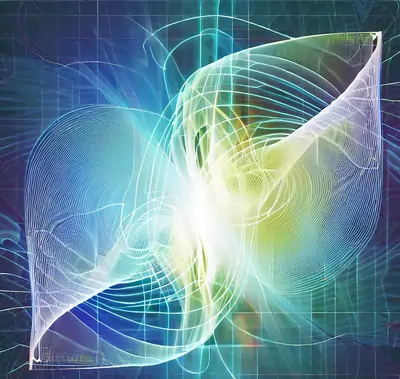
Mathematically, the Uncertainty Principle can be expressed as:
Δx * Δp ≥ ħ / 2
Where:
- Δx represents the uncertainty in position.
- Δp represents the uncertainty in momentum.
- ħ (pronounced "h-bar") is the reduced Planck constant, a fundamental constant of nature.
This principle fundamentally challenges our classical intuitions about measurement and predictability. It tells us that at the quantum level, there are fundamental limits to what we can know about particles. But how does this concept, rooted in the microcosm of quantum physics, relate to the macroscopic fields of harmonic analysis and signal processing? Let's explore.
Uncertainty Principle in Harmonic Analysis
The Uncertainty Principle in Harmonic Analysis is a fundamental concept in mathematics and signal processing. It asserts that there is a fundamental limit to how precisely we can simultaneously measure a function and its Fourier transform.This principle has profound implications in signal processing, quantum mechanics, and data analysis, highlighting the fundamental trade-off between accuracy and frequency resolution.
Fourier Transform and Heisenberg's Uncertainty Principle
In harmonic analysis, the Fourier Transform is a fundamental tool used to decompose complex signals into their constituent sinusoidal components. It allows us to represent a signal in the frequency domain, revealing the different frequency components that make up the signal.
The Uncertainty Principle finds a natural home in harmonic analysis when we consider the trade-off between time and frequency precision. If we narrow down our focus on a specific time interval in a signal (reducing Δt, the uncertainty in time), the corresponding frequency representation (Δf, the uncertainty in frequency) will spread out. Conversely, if we aim to precisely determine the frequency components (reducing Δf), the temporal information will become less precise (increasing Δt). This trade-off mirrors Heisenberg's Uncertainty Principle.
Mathematically, this relationship can be expressed as:
Δt * Δf ≥ 1 / (4π)
This inequality signifies that there is a limit to how well we can simultaneously pinpoint the temporal and spectral characteristics of a signal. The narrower the time window, the broader the frequency spectrum, and vice versa.
Windowed Fourier Transforms
One practical application of the Uncertainty Principle in harmonic analysis is evident in the use of windowed Fourier transforms. In real-world scenarios, we often deal with signals that are non-stationary, meaning their spectral content evolves over time. To analyze such signals, we use short-time Fourier transforms (STFT) or wavelet transforms, both of which involve windowing the signal and performing Fourier analysis within these windows.
The choice of window size directly impacts the trade-off between time and frequency precision. A smaller window provides better temporal localization but sacrifices frequency precision, while a larger window offers better frequency localization but loses temporal accuracy. This windowing process embodies the essence of the Uncertainty Principle, as we are constrained by the same fundamental limit.
Gabor Transform: Balancing Time and Frequency
The Gabor Transform is a specific instance of a windowed Fourier transform that seeks to strike a balance between time and frequency precision. It utilizes a Gaussian window, which minimizes the uncertainty product Δt * Δf while still providing a reasonable compromise between the two.
The Gabor Transform is particularly useful in applications like audio processing, where both temporal and spectral details are crucial. It enables us to extract essential information from signals while respecting the limitations imposed by the Uncertainty Principle.
Uncertainty in Signal Processing
Uncertainty in signal processing is a fundamental challenge that arises from noise, measurement limitations, and imperfect data. Understanding and quantifying uncertainty allows for robust decision-making in fields like telecommunications, image processing, and autonomous systems. By addressing uncertainty, signal processing techniques can enhance the reliability and accuracy of data analysis, ultimately leading to improved outcomes in diverse applications.
Sampling and Reconstruction
In signal processing, one of the central challenges is sampling continuous signals for digital processing and later reconstructing them accurately. The Nyquist-Shannon sampling theorem, a cornerstone of signal processing, tells us that to faithfully represent a signal, we must sample it at a rate greater than twice its highest frequency component.
This theorem embodies an uncertainty principle of its own. If we want to preserve all frequency information in a signal, we must capture it at a high sampling rate (reducing Δt, the sampling interval). However, this also means that our time-domain representation becomes densely sampled, potentially leading to computational challenges and storage requirements. Conversely, reducing the sampling rate to ease computational burdens results in aliasing, effectively limiting our ability to accurately reconstruct the signal in both time and frequency domains.
Windowing in Signal Processing
Just as in harmonic analysis, windowing plays a crucial role in signal processing. When analyzing finite segments of a signal, we often employ window functions to limit the scope of our analysis. Common window functions include the Hamming, Hanning, and Blackman windows, each with its trade-offs between time and frequency precision.
The choice of window function and its size can significantly impact the outcome of signal processing tasks. A narrower window provides better time resolution but broadens the frequency spectrum, while a wider window enhances frequency precision but blurs temporal details. This windowing process echoes the Uncertainty Principle's influence on signal processing, emphasizing the delicate balance between time and frequency precision.
Applications and Implications
The Uncertainty Principle, a cornerstone of quantum mechanics, has far-reaching applications and profound implications across various scientific disciplines. Although initially formulated in the context of quantum physics, its influence extends well beyond the quantum realm. In this article, we will explore some of the key applications and implications of the Uncertainty Principle in fields such as quantum mechanics, chemistry, technology, and philosophy.
Quantum Signal Processing
The intersection of quantum mechanics and signal processing has given rise to a burgeoning field known as Quantum Signal Processing (QSP). QSP aims to harness the principles of quantum mechanics to process and manipulate quantum information efficiently. Despite its seemingly abstract nature, QSP has practical implications that could revolutionize various aspects of computation, communication, and cryptography.
Leveraging Quantum Uncertainty
At the heart of QSP lies the Uncertainty Principle, a fundamental concept in quantum mechanics. In the context of quantum signals and information, this principle manifests as a fundamental limit on the precision with which we can simultaneously measure certain properties of quantum systems. For instance, if we want to precisely determine a quantum system's energy, we may have limited knowledge of its exact time evolution, and vice versa. This inherent trade-off aligns with the broader principles of signal processing.
Quantum Algorithms
One of the most exciting aspects of QSP is the development of quantum algorithms that leverage quantum properties to solve problems faster and more efficiently than classical counterparts. Quantum algorithms like Shor's algorithm and Grover's algorithm have demonstrated the potential to factor large numbers and search unsorted databases exponentially faster than classical algorithms. These algorithms often rely on exploiting the quantum Uncertainty Principle in novel ways, enabling quantum computers to process information that would be intractable for classical computers.
Quantum Communication
In the realm of quantum communication, the Uncertainty Principle plays a critical role in the security of quantum key distribution (QKD) protocols such as the famous BB84 protocol. These protocols exploit the fundamental uncertainty associated with quantum measurements to detect eavesdropping attempts. If an eavesdropper tries to intercept quantum-encoded information, the act of measurement unavoidably disturbs the quantum state, alerting the legitimate users to potential security breaches.
Quantum Cryptography
Quantum cryptography takes advantage of the Uncertainty Principle to achieve ultra-secure communication. For example, quantum key distribution systems use entangled particles, such as photons, to transmit cryptographic keys. Any attempt to eavesdrop on these particles inevitably disrupts their quantum states, allowing the parties involved to detect the intrusion. This ensures a level of security that is theoretically unbreakable, thanks to the fundamental limitations imposed by the Uncertainty Principle.
Time-Frequency Analysis
In classical signal processing, the influence of the Uncertainty Principle is particularly pronounced in the domain of time-frequency analysis. This field encompasses a wide range of techniques used in various applications, including speech processing, image processing, and radar systems. The fundamental challenge in time-frequency analysis is achieving a balance between temporal precision and spectral accuracy.
Speech Processing
In speech recognition and analysis, understanding the dynamics of phonemes and their transitions is crucial. To capture these rapid changes in speech, a small time window is necessary. However, this fine temporal resolution comes at the cost of frequency precision. As a result, the spectral information may appear blurred, making it more challenging to distinguish between similar phonetic sounds.
Image Processing
Image processing often involves the analysis of images in both the spatial and frequency domains. When applying Fourier-based transformations or wavelet analysis, the choice of window size determines the trade-off between fine spatial detail and accurate frequency characterization. Smaller windows excel at preserving sharp spatial features but may lose information about the image's broader frequency content.
Radar Systems
In radar systems used for target detection and tracking, time-frequency analysis helps discern moving objects amidst cluttered environments. Here, a balance between time and frequency precision is crucial. A narrow time window can reveal the precise location of a target but may miss slower-moving objects with lower frequencies. Conversely, a broader time window provides better frequency information but sacrifices the ability to precisely locate fast-moving targets.
Music Analysis
In music analysis, especially for tasks like instrument recognition or pitch detection, time-frequency analysis techniques such as the Short-Time Fourier Transform (STFT) or the Continuous Wavelet Transform (CWT) are employed. Musicians and audio engineers use these methods to extract information about the spectral content of musical notes, harmonics, and transients. The choice of analysis window impacts the ability to accurately distinguish between different musical elements.
Striking the Right Balance
Engineers and scientists in these domains must make informed decisions about the parameters and techniques they use for time-frequency analysis. This includes choosing appropriate window functions, window sizes, and overlapping windows to strike the right balance between temporal and spectral precision based on the specific requirements of their applications. Achieving this balance is essential for extracting meaningful information from signals and making accurate decisions in various fields.
The Uncertainty Principle, rooted in quantum mechanics, has a profound impact on both Quantum Signal Processing and classical Time-Frequency Analysis. Whether in the quest for quantum algorithms or in the practical tasks of speech recognition, image processing, radar systems, and music analysis, the delicate interplay between time and frequency precision is a constant consideration. Recognizing and working within the bounds set by the Uncertainty Principle empowers scientists and engineers to push the boundaries of what's possible in these exciting fields of study and application.
Conclusion
The Uncertainty Principle, born in the realm of quantum mechanics, has far-reaching implications that extend into the domains of harmonic analysis and signal processing. Whether we are analyzing the spectral content of a musical composition, processing audio signals, or designing quantum algorithms, the trade-off between time and frequency precision is a fundamental consideration.
As researchers and practitioners in these fields continue to push the boundaries of what is possible, they must navigate the constraints imposed by the Uncertainty Principle with creativity and ingenuity. By doing so, we unlock new insights into the nature of signals, both classical and quantum, and advance our understanding of the universe, one uncertainty principle at a time.